
a quadrupedal robot that can walk in the dark? (with videos)
[ad_1]
(Nanowerk News) A team of Korean engineering researchers has developed a quadrupedal robotic technology that can climb up and down stairs and move around without falling over uneven environments such as tree roots without the aid of visual sensors or touch even in disaster situations where visual confirmation is required due to darkness or smoke thick of flames.
KAIST announced that Professor Hyun Myung’s research team at the Laboratory of Urban Robotics at the School of Electrical Engineering developed a walking robot control technology that enables powerful ‘blind locomotion’ in a variety of unconventional environments.
The KAIST research team developed the “DreamWaQ” technology, which is so named because it allows a walking robot to move around even in the dark, just as a person can walk without visual assistance when getting out of bed and going to the bathroom in the dark. With this technology mounted on top of any legged robot, it is possible to create many different types of “DreamWaQers”.
(embed)https://www.youtube.com/watch?v=JC1_bnTxPiQ(/embed)
Existing walking robot controllers are based on kinematics and/or dynamics models. This is stated as a model-based control method. In particular, in atypical environments such as open and uneven terrain, terrain feature information needs to be obtained more quickly to maintain walking stability. However, it has been shown to rely heavily on cognitive abilities to survey the surroundings.
In contrast, the controller developed by Professor Hyun Myung’s research team based on the deep reinforcement learning (RL) method can quickly calculate the appropriate control commands for each walking robot motor through data from various environments obtained from the simulator. While the existing controller learned from the simulation requires its own re-orchestration in order to work with the actual robot, it is expected that the controller developed by the research team can be easily applied to various walking robots because it does not require additional tuning processes.
DreamWaQ, the controller developed by the research team, mainly consists of a context estimation network that estimates ground information and a robot and policy network that calculates control commands. The context-assisted estimator network estimates basic information implicitly and robot state explicitly through inertial and shared information. This information is fed into the policy network which will be used to generate optimal control commands. The two networks are studied together in the simulation.
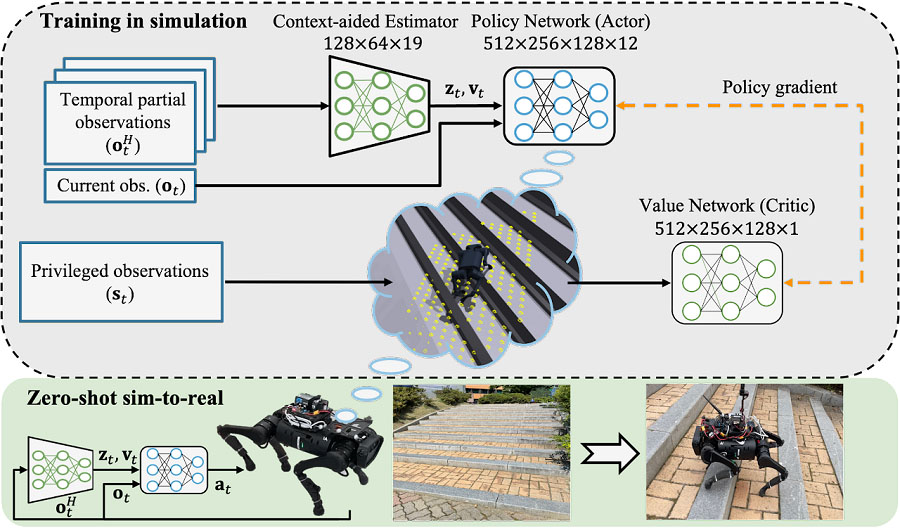
While context-assisted estimator networks are studied through supervised learning, policy networks are studied through actor-critic architecture, a deep RL methodology. The actor network can only implicitly infer the surrounding field information. In the simulation, the surrounding terrain information is known, and the critique, or value network, which has the appropriate field information evaluates the actor’s network policies.
This whole learning process takes only about an hour on a GPU-enabled PC, and the actual robot is only equipped with a network of actors being learned. Without looking at the surrounding terrain, he goes through the process of imagining which environment is similar to one of the various environments studied in the simulation using only the inertial sensor (IMU) inside the robot and measurement of joint angles. If it suddenly encounters an offset, such as a ladder, it won’t know it until its feet touch the steps, but it will quickly assemble terrain information the moment its feet touch the ground. Then control commands matched to approximate terrain information are transmitted to each motor, allowing running to be quickly adjusted.
The DreamWaQer robot walks not only in a laboratory setting, but also in an outdoor environment around a campus with lots of sidewalks and speed bumps, and over a field with lots of tree roots and gravel, demonstrating its ability by overcoming stairs by one height which is two-thirds of its body. . Moreover, regardless of its environment, the research team confirmed that it was able to run stably from a slow speed of 0.3 m/s to a rather fast speed of 1.0 m/s.
The results of this study (“DreamWaQ: Learning Powerful Quadrupedal Locomotion with Implied Terrain Imagination through Deep Reinforcement Learning”) which was produced by a doctoral student, I Made Aswin Nahrendra, as the first author, and his colleague Byeongho Yu as co-author. It has been accepted for presentation at the upcoming IEEE International Conference on Robotics and Automation (ICRA) which is scheduled to be held in London at the end of May.
[ad_2]
Source link